![[object Object]](/_next/image?url=https%3A%2F%2Fcdn.sanity.io%2Fimages%2Fei1axngy%2Fproduction%2Fef070923b464a8de88e1f86200d4ebb6f0a60c57-474x473.webp&w=256&q=75)
by The digiLab Team
Updated 25 April 2024
digiLab and PSFC hold Joint Workshop on Machine Learning for Fusion Energy
![[object Object]](/_next/image?url=https%3A%2F%2Fcdn.sanity.io%2Fimages%2Fei1axngy%2Fproduction%2Fbe286068881bc9e2cda58878e81247f3ecd596b3-1280x720.png&w=2048&q=75)
digiLab and PSFC hold Joint Workshop on Machine Learning for Fusion Energy
The Massachusetts Institute of Technology’s Plasma Science and Fusion Centre (PSFC) held a collaborative workshop with digiLab, a machine learning (ML) company, to explore the advantages of probabilistic machine learning in advancing the development of fusion power. The full-day workshop was led by digiLab’s CEO and Turing AI Fellow, Professor Tim Dodwell, digiLab’s Fusion Team, and attended by members of PSFC.
Professor Dodwell said,
“The collaboration between MIT PSFC and digilab is a great opportunity for cross-fertilisation. It is essential for experts in machine learning [ML] and fusion science to collaborate in order to unlock the advances of the rapidly developing ML landscape. Utilising explainable ML in R&D will both accelerate and de-risk the development of fusion energy.”
The day kicked off with a deep dive into advanced probabilistic ML methods called Gaussian Processes (GPs). GPs help researchers and engineers acquire the next best data point in their simulation or carry out the next best test in their experiment to optimise the research process. It also allows researchers to know how confident the model is in its output, enabling better decision making and confidence in deployment.

IMG-20240424-WA0003
Professor Tim Dodwell at the PSFC-digiLab collaborative workshop.
Together representatives from digiLab and PSFC explored the opportunities for machine learning and AI in plasma modelling and control, as well as material and fuel cycle research. As fusion progresses further towards operational power-plants, it is vital to optimise fusion plasmas to get the most energy out from what is put in. To do this, simulations of core plasma turbulence can be leveraged, but these simulations are often computationally expensive. ML can help speed up the complex simulations to predict and optimise the core performance.
The control of plasma will be important to maintain good fusion performance and uptime, but also to ensure harmful events are avoided. PSFC and digiLab discussed the use of AI for the prediction and avoidance of harmful events such as disruptions. Central to the discussion was the need to create trustable, explainable algorithms, if they are to be used to control real fusion power-plants. digiLab and PSFC are gaining this trust through quantifying uncertainties associated with models and by developing methods to explain why an AI algorithm makes certain decisions.
The latter parts of the workshop focused on applying Gaussian Processes to model how fusion fuel moves through certain materials. In fusion, the fuel tritium is a relatively rare substance that is also radioactive. To ensure a fusion plant is self-sustaining and highly safe, it is vital to understand how tritium is created, and how it will move through future devices.
Though there was ample cross-pollination and problem solving at the workshop, all of fusion’s challenges weren’t solved in a day. There is no shortage of potential applications of machine learning and AI in fusion, and the experts at PSFC and digiLab will continue to push the envelope in the future. Amongst the various tools used by both institutions, collaboration and knowledge sharing is one of the most vital.
Interested in partnering or collaborating with digiLab to accelerate the commercialisation of fusion energy? Get in touch at fusion@digilab.co.uk
Featured Posts
If you found this post helpful, you might enjoy some of these other news updates.
The Ethical AI Database
digiLab is part of the Ethical AI Database

Dr Andy Corbett
Understanding Uncertainty Quantification: The Different Types
Understanding Uncertainty Quantification - an article on the different types.
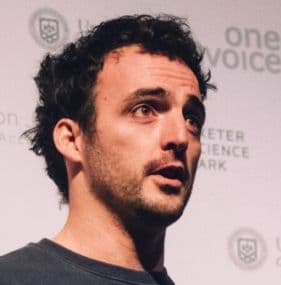
Prof Tim Dodwell
Tech Nation Rising Stars 5.0 - delighted national winners!
Tech Nation Rising Stars 5.0 winners

The digiLab Team